Globally, the health burdens of tuberculosis (TB) and HIV are extremely high. TB is the second highest cause of infectious-disease deaths (behind COVID-19) and is the leading cause of death for individuals with HIV. While progress in detection, treatment, and prevention has been improved for both, declines in incidence are not at the levels required to end them as major public health threats. To help accelerate incidence declines, research teams at IDM focus on developing and applying advanced modeling techniques for epidemic modeling, data analysis, and genomic epidemiology. Our models are used to design and optimize interventions, such as new vaccines, diagnostics, and innovative prevention approaches targeting upstream determinants of high-risk groups (such as nutrition and housing for TB or addressing heterogeneity in HIV risk). We collaborate with global, academic, and in-country partners to translate research into actionable outcomes.
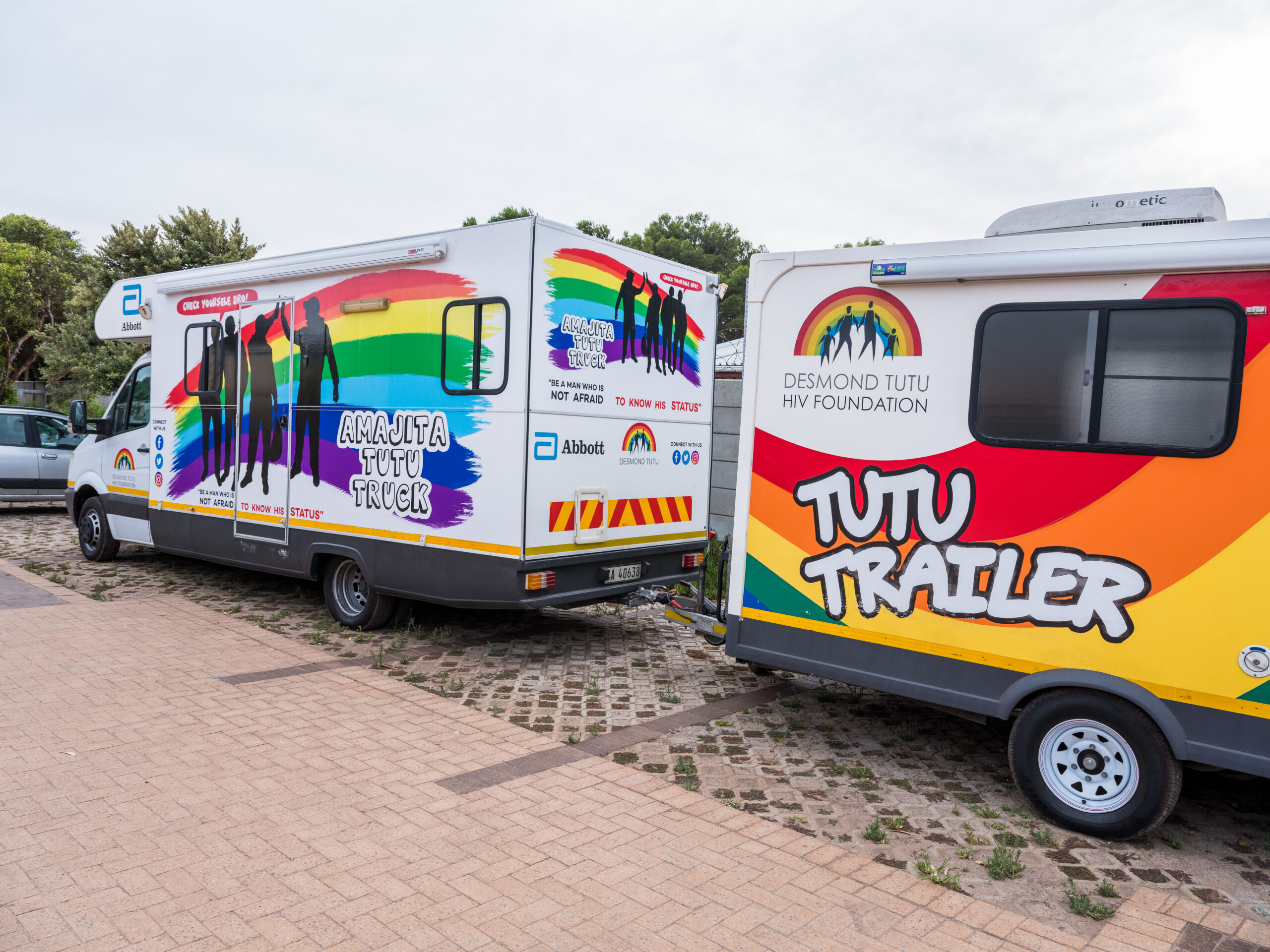
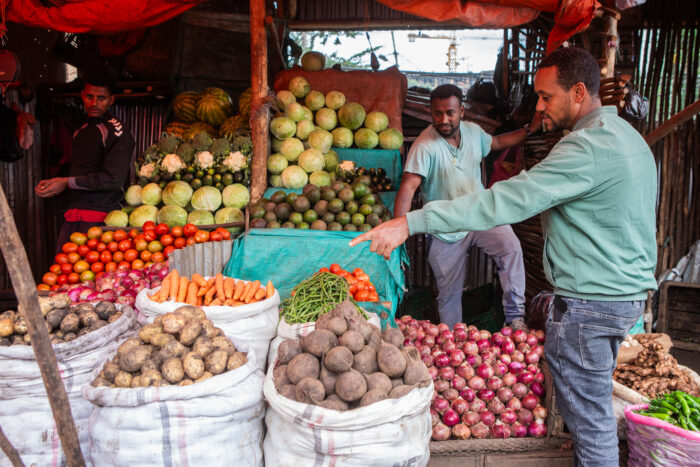
Our mission
The team is dedicated to:
- Accelerating TB burden reduction by identifying key drivers of transmission, such as subclinical TB and poor nutrition, to inform targeted interventions.
- Developing interventions that address both biological and social determinants of TB with programmatic partners.
- Enhancing the design and interpretation of studies and trials of novel TB control strategies.
- Estimating the potential impact of HIV prevention tools, including pre-exposure prophylaxis (PrEP), ART, and vaccines.
- Accelerating HIV incidence reduction by identifying key drivers of transmission, focused on specific geographic, demographic, or behavioral characteristics, to inform targeted interventions.
Our approach
Our modeling approach encompasses several techniques:
- Developing and using agent-based models to simulate the natural history and epidemiology of TB or HIV
- Running simulations on clinical trial data to predict and interpret outcomes for novel TB or HIV interventions
- Creating branching process model simulations to produce HIV phylogenies for downstream analysis
- Developing causal inference techniques to derive insights from longitudinal data and identify novel targets for intervention or policy
- Utilizing statistical modeling and small-area estimation to understand local TB or HIV epidemiology
- Integration of preclinical, clinical, and epidemiological data to synthesize knowledge across scales and inform the design of intervention strategies
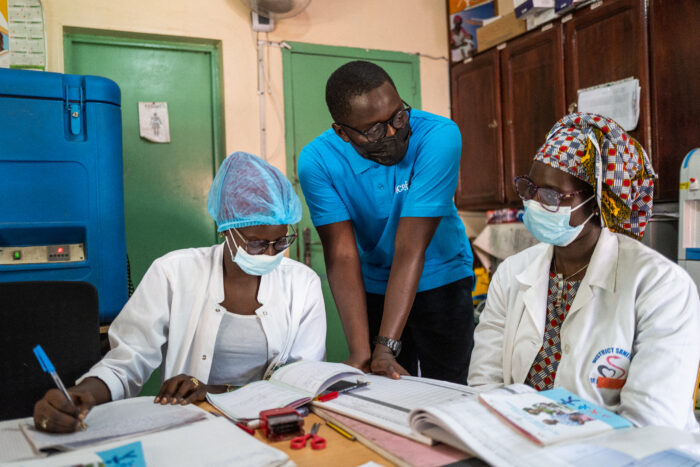
Publications
To learn more about IDM’s work on this topic, browse our publications!
Code and tools
Software developed by IDM is freely available; you are encouraged to try out the tools developed for use on this topic!
View all code and tools